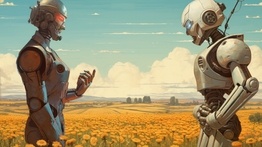
Comparing Large Language Models
Different language models have different capabilities, and different approaches yield very different results. Understanding the limitations and how these models are trained will be increasingly important, especially as it comes time to navigate which tools to use, when and how.